10 Best AI models you should definitely know about (and why they matter)
As we continue to use more AI in our workflows, it becomes important to have a list of best AI models that can be used for specific tasks. In this article, we will talk about general AI models, LLMs, and more that you can use to build AI-driven applications.
Do you know all the LLMs that OpenAI, Anthropic, X AI, and Google support? It’s hard to keep track, right? AI updates are moving fast, not just with models, but also with new methodologies like Model Context Protocol (MCP).
You cannot skip a day of learning if you want to stay updated with all AI-related knowledge – whether it's new models, smarter tools, or figuring out the best infrastructure for deploying AI models.
How I selected the models in this list
In this article, I listed 10 AI models that you can use to build AI-powered applications.
These recommendations come from personal experience (I will also list use cases so you can better understand when to use each one), research papers, articles on models that achieved the best performance for certain tasks (like YOLO for computer vision), some lesser know Reddit threads (which often acts as a gold mine of information to me) and Peter Yang’s famous article on “An Opinionated Guide on Which AI Model to Use in 2025”.
When it comes to models, we are spoiled with choices now (Even while writing this article, I learnt about Llama 4 being launched).
I personally use 2-3 different models for different types of tasks.
My go-to ones are Claude 3.7 Sonnet for coding and 4o for creative tasks such as writing. Along with these, I also use tools like v0/Bolt to build the frontend, Pieces for help within the IDE, and acting as a second brain for me.
While I cannot cover everything AI-related in this one blog, I will list some of the best Gen AI models that you should know about and also cover how you can use them in your daily tasks.
What are the different types of AI models?
Before we get into the top 10 models, let us see what the different types of AI models are (It is easy to think models = LLM, since that is the talk of the town now, but there’s more to AI than large language models).
LLMs: LLMs are something that we use almost every day. They are a specific type of generative model that excels in text and is used for generating human-like text, content creation, and text analysis. Examples of LLMs can be the GPT series, Gemini, etc.
Generative Models: GenAI models, compared to LLMs, are designed to create new content that resembles their training data. They are used to create new content (text, images, audio, video) based on patterns in training data. DALL-E and Sora are some examples.
Computer Vision Models: If you drive a car and you break a traffi rule, you get a ticket on your email. This is a classic example of a computer vision model in use where the car number is processed, then used specialized algorithms to identify and read license plates. After human verification, tickets are typically sent through official mail channels. A model used for that is YOLO.
Recommendation Systems: We have seen recommendation systems almost everywhere, from e-commerce websites like Amazon to YouTube. YouTube's Neural Network Recommender is a great example of a recommendation system where you get music suggested based on what you played last.
Time Series Models: These analyze and predict sequential data patterns over time. An example of this would be DeepAR by Amazon.
Reinforcement Learning Models: Reinforcement learning models learn actions through trial and error. If you remember how DeepMind's AlphaGo made history by defeating world champion Go players, that is an example of reinforcement learning.
Graph Neural Networks: These process data represented as graphs with nodes and edges. DeepWal is an example of a GNN.
GANs: GANs generate realistic synthetic data through adversarial training between generator and discriminator networks. Pix2Pix is a popular example of a GAN.
Transformer Models: GPT is powered by transformer models. It works by breaking down the prompt into tokens, then embedding it and going through encoders and decoders to generate a result.
Decision Tree Models: For those who have played around with machine learning before generative AI, you might already be aware of XGBoost, which is an example of a decision tree model where predictions are made by following a tree-like structure of decisions and outcomes.
Here’s the top 10 models that I think you should know about (if you don’t know already)
We are either building with AI or with the help of AI. I personally use it to code proof-of-concepts a lot and sometimes also use it for my silly side projects.
So I usually look for models that can be my copilot and can also be used for integration. I have a long-pending side project that is all about plants, including identifying them, determining their types, understanding the soil type, and checking for diseases.
This project led me to research models I could use, such as LLMs, object detection models, and more.
I experimented with many of them, and below are the top 10 models on my list.
GPT-4o
If you use ChatGPT for any task, you have probably already used GPT-4o. Before I talk about what I like and don’t like about 4o, I should mention its image generation capabilities.
The internet was flooded with beautiful Ghibli images, and I also saw people creating YouTube thumbnails, banners, and much more.
Here’s an example of a person extracting assets using 4o.

This shows how much image generation with AI has improved. You can learn more about the 4o image generation capabilities in their announcement article.
The image generation capability has definitely made 4o rank higher in my list of go-to AI models. I also used GPT-4o in my side projects using the API, so if you are planning to build with GPT-4o, going by the API route is a good idea.
When OpenAI launched GPT-4o, they said it is better at vision and audio understanding compared to existing models, while also being much faster and 50% cheaper in the API. When compared to GPT 4, which has an 8k context window, GPT 4o has 128k token context window, so 4o is better at memory and has more context.
Here’s an evaluation comparing all GPT models:
Model | Prompt | MMLU | GPQA | MATH | HumanEval | MGSM | DROP (F1, 3-shot) |
---|---|---|---|---|---|---|---|
OPENAI GPT 4s | |||||||
gpt-4o | chatgpt | 88.7 | 49.9 | 76.6 | 92.0 | 90.5 | 83.4 |
gpt-4o | assistant | 87.2 | 49.9 | 76.6 | 91.0 | 89.9 | 83.7 |
gpt-4-turbo-2024-04-09 | chatgpt | 86.5 | 49.1 | 72.2 | 87.6 | 88.6 | 85.4 |
gpt-4-turbo-2024-04-09 | assistant | 86.7 | 49.3 | 73.4 | 88.2 | 89.6 | 86.0 |
gpt-4-1106(-vision)-preview | chatgpt | 84.6 | 42.1 | 64.1 | 82.2 | 86.5 | 81.3 |
gpt-4-1106(-vision)-preview | assistant | 84.7 | 42.5 | 64.3 | 83.7 | 87.1 | 83.2 |
gpt-4-0125-preview | chatgpt | 84.8 | 39.7 | 64.2 | 88.2 | 83.7 | 83.4 |
gpt-4-0125-preview | assistant | 85.4 | 41.4 | 64.5 | 86.6 | 85.1 | 81.5 |
Pros: It is multimodal (can work with different types of data), fast, cost-effective, and has a high level of accuracy.
Cons: It still hallucinates a bit and has difficulty following instructions. I have seen this especially in coding-related tasks; when I prompt it to take a different approach, it doesn’t, instead, it builds upon the existing solution.
I would suggest you use 4o for more creative tasks instead of complex tasks such as coding. I mostly use it for writing, as shown in the image below.

Using the GPT-4o model within Pieces to generate a technical article on load balancing.
Claude 3.7 Sonnet
As a developer, Claude 3.7 Sonnet is my go-to model for coding-related tasks. It’s just not me saying it, but devs on Reddit forums are also impressed by 3.7 Sonnet (When Reddit says something is good, you know it is good)

The screenshot above has been taken from this Reddit thread.
"Claude 3.7 Sonnet is the first hybrid reasoning model and our most intelligent model to date. It’s state-of-the-art for coding and delivers significant improvements in content generation, data analysis, and planning."
— Anthropic, on the launch of Claude 3.7 Sonnet
What I like about Claude (from 3.5 Sonnet) is the ability to use Claude via the API and direct it to use computers the way people do – by looking at a screen, moving a cursor, clicking buttons, and typing text.
Not just for computer use, but you can also use the APIs to build applications of your choice (Like I built this tutorial generator using 3.5 Sonnet).
Claude has been used in general as well as extended thinking mode, where it can be used to solve more complex problems in a step-by-step manner, and when benchmarked with other models, this is how it performs:

The image above has been taken from Anthropic’s Claude 3.7 Sonnet announcement blog.
Pros: Other than coding, what I really like about Claude 3.7 Sonnet is its ability to extract information from visuals like charts, graphs, and complex diagrams for data analysis.
Cons: While it calls itself a general-purpose model, I haven’t found it very helpful in writing-related tasks. In my opinion, GPT 4o with search mode works much better for writing and creative tasks, while Claude 3.7 Sonnet makes many logical mistakes and sometimes gives inaccurate responses.

Screenshot of what a Reddit user had to say about its writing ability.
💡If I were you, I would choose 3.7 Sonnet for coding (especially frontend) and not choose it for anything related to creativity or soft skills. Pieces supports this model among many others, so I’d give it a try to run on your OS, especially after they integrated MCP.
YOLO (You Only Look Once)
If you’ve ever tried object detection in real time, there’s a good chance you’ve used a version of YOLO.
I remember using it for sign language detection a few years ago, and a friend of mine used it to play air drums.
Whether for fun side projects like these or serious production-level applications like traffic monitoring, YOLO has been the model for anything object detection related.
This is mostly because of its ability to identify and localize multiple objects in images swiftly and its one-shot architecture.
YOLO does everything in a single neural network pass, dividing them into regions and predicting bounding boxes and class probabilities simultaneously, instead of running in multiple steps (thus the name "You Only Look Once").
This makes it super fast, even on devices like Raspberry Pi or mobile phones.
YOLOv8 (by Ultralytics) supports not just object detection but also instance segmentation, pose estimation, and multi-object tracking – so it's not just good at telling what’s in an image, but also where and how.
I can already think of great cases for physical activity-related apps.
You can use YOLOv8 in your apps via the Ultralytics Python library or even directly in-browser with ONNX.js or TensorFlow.js models. Here’s a quick way to use YOLOv8 in Python:

Screenshot of using Pieces Copilot to learn about YOLO.
Pros: Fast, lightweight, real-time, good accuracy, supports training on custom datasets, and works on most platforms (including mobile + edge devices).
Cons: Can struggle with small objects, overlapping instances, and doesn’t always perform well in low-light or noisy environments. Training also requires a bit of computation if you're going custom.
If you’re building anything that needs to "see" the world around it – security cams, retail store analytics, autonomous bots, or even fun side projects – YOLO is probably the easiest and fastest way to get started.
BERT
A few years back, my favorite projects were the ones on sentiment analysis.
I still remember building a Twitter sentiment analysis tool back then. If you have played around with NLP, there’s a high chance that you have come across BERT.
Most models before BERT used to process sentences one word at a time, but BERT could read in both directions at once, which was great for more context.
Google started using BERT in Search to improve how it interprets queries, and since then it’s popped up everywhere – from chatbots and smart assistants to internal tools at companies like Microsoft and Amazon.
If you would like to build similar systems/applications with BERT, you don’t need to train it from scratch and can instead use a pre-trained version from Hugging Fac, as shown in the image below.

Screenshot of using Pieces Copilot to learn about BERT.
Pros: BERT has high accuracy for many NLP tasks and requires less training time. The best part is that it is available for free and can be used with platforms like Hugging Face.
Cons: Unlike LLMs, BERT has limited context understanding and is not ideal for text generation, especially when compared to something like GPT-4.
If you were to build for tasks like search, text classification, or NLP, BERT is a good model to consider.
LLaMA
You might’ve seen a bunch of open-source LLMs being released recently, and most of them are based on LLaMA in some way.
LLaMA (by Meta) was basically Meta’s answer to GPT-style models, and when it got leaked (yep, that happened), the open-source AI scene exploded.
Suddenly, people were fine-tuning LLaMA models on personal laptops and using them in all kinds of local apps. That leak might’ve been chaotic, but it seriously helped the open-source community level up fast.
Just 2 days back, they also released LlaMA 4 here, which will enable people to build more personalized multimodal experiences.
What makes LLaMA interesting is that it was trained to be super efficient. Even the smaller versions (like LLaMA 7B) perform surprisingly well for their size.
Meta also put in a lot of work to make them competitive with GPT-3.5 level performance, and in some benchmarks, they even beat it.
Here’s a comparison of the latest LlaMA model with other models:

The above picture has been taken from the Meta announcement blog where it benchmarks Llama 4 with other models.
You'll see LLaMA-based models powering things like private GPT-style chat apps (e.g., LM Studio or Ollama), coding assistants, and even full-blown local copilots.
If you want to run your own AI model offline or on your own server, this is one of the best options right now.
Here's how you can run LLaMA using Ollama libraries in Python. And if you activate long-term memory, it brings context awareness to your old projects:

Screenshot of using Pieces Copilot to learn how to use Ollama to run Llama models.
Pros: The biggest perk of LLaMA is that it is open-source and the ease of using it locally (in case you want more security) with offline capabilities.
Cons: The previous models of LLaMA were not as good as GPT-4 or other models in reasoning and other tasks. Though they say that the latest model is great, here's what a dev on X has to say:

Whisper
If you’ve ever tried to transcribe audio using AI, Whisper is probably the model that gave you scarily accurate results.
What makes it cool is that it’s trained on a massive amount of multilingual and multitask data, so it doesn’t just work for English; it handles multiple languages and accents really well.
I’ve seen people use Whisper to transcribe podcasts, YouTube videos, build voice assistants, and even create meeting notes from Zoom calls.
Some folks even use it to process real-time audio streams. It’s that versatile.
What I like is that Whisper just works out of the box. No extra fine-tuning needed.
You can plug it into your app using OpenAI’s Whisper API or run it locally using whisper.cpp (which is optimized to work on CPUs).
You can also use it with tools like Groq. And if you’re using Python, it’s super easy to get started with the original OpenAI model:

Screenshot of using Pieces Copilot to learn how to get started with Whisper using Python.
There’s also a web UI version that runs locally and gives you a simple interface to upload files and get clean transcriptions.
Pros: Accurate, multilingual, open-source, can run locally, handles noisy audio better than most models.
Cons: Slower on CPU (unless you use optimized versions like whisper.cpp), doesn’t do speaker diarization (who said what), and outputs raw text (you’ll need to add timestamps or formatting yourself if needed).
➡️ If you're building anything that involves voice, like a podcast tool, video editor, or even a voice note app, Whisper can be your choice to get started.
XGBoost
I come from that era of Machine Learning where we needed to learn math first and then learn how to use different libraries (within Python/R).
Since then, XGBoost has been a staple for regression and classification problems, as well as ranking and user-defined prediction tasks.
There’s a reason why XGBoost keeps showing up in machine learning projects, hackathons, and even high-stakes production systems.
❕It’s fast, reliable, and good at working with structured (tabular) data.
XGBoost is used in places like credit scoring, fraud detection, and churn prediction, along with companies like Airbnb, PayPal, and even banks that still use it under the hood.
What makes it powerful is its ability to build trees sequentially, each one trying to fix the mistakes of the previous one.
And thanks to smart regularization and pruning techniques, it avoids overfitting better than a lot of similar models. You don’t need a giant dataset or a GPU to get good results either.
Here’s a tutorial on how to train an XGBoost model in Python.
If you want to skip the tutorial and get your hands dirty with code directly, you can use tools like Pieces within your IDE or standalone to learn the basics, like I did here:

Screenshot of using Pieces Copilot to learn how to use XGboost model in Python.
Pros: One big perk of XGBoost is that it is open-source and maintained by researchers/ML engineers, and it has a built-in cross-validation method that helps in improving model accuracy.
Cons: Doesn’t work well on images or text, can overfit if you push it too hard, and large datasets can slow it down without GPU support.
💭For structured data tasks that need real-world performance without a ton of infrastructure, XGBoost is still one of the best options out there. I usually reach for it when I want quick, clean results and don’t want to mess with deep learning.
Stable Diffusion
Stable Diffusion changed the game for image generation, especially for people who wanted to create high-quality visuals without needing access to a powerful cloud setup.
Unlike earlier diffusion models that required massive resources, this one is optimized to run on consumer GPUs. That means you can generate photorealistic images from text prompts locally, on your own machine.
It’s an open-source model released by Stability AI and can be run on your local machine (and considered one of the best AI open source models in Reddit forums).
People use it to make everything from concept art and avatars to wallpapers, comic book panels, and even product mockups. It’s also being used in apps like Leonardo.Ai, and InvokeAI.
The most recent version, Stable Diffusion 3.5 made things even better with a new Multimodal Diffusion Transformer (MMDiT) backbone.
It’s more reliable with complex prompts, generates cleaner outputs, and introduces Query-Key Normalization (QKN) for better stability during training. You also get more variety in styles, like 3D, illustration, line art, and photography.
Stable Diffusion works by gradually denoising a random noise image guided by the text prompt, and the results are seriously impressive.
Want a "cyberpunk astronaut riding a dragon"? It’s just a prompt away.
You can even fine-tune it with your own images using tools like DreamBooth or LoRA for custom generations.
Here’s how you can run it locally using the popular diffusers library by Hugging Face:

Screenshot of using Pieces Copilot to learn how to use Stable Diffusion.
There’s also AUTOMATIC1111’s WebUI for Stable Diffusion, which gives you a beautiful local interface with control over settings like image size, steps, seed, and more, without writing any code.
Pros: Open-source, highly customizable, runs locally, now more stable and detailed with 3.5, works with multiple styles, massive ecosystem of extensions and models.
Cons: Still needs a decent GPU for good performance, prompt crafting matters a lot, and the setup (especially for advanced models or fine-tuning) can be overwhelming at first.
If you want to experiment with a model to generate creatives like visuals for blog posts, thumbnails, or just want to explore creative ideas, give Stable Diffusion a try (at least until the 4.0 image generation API is out 😉).
Mistral 7B
Mistral 7B is one of those models that hits the sweet spot between speed and quality. It’s a dense 7B parameter model, so no Mixture of Experts stuff here, and it performs surprisingly well for its size.
What really makes it stand out is how fast and efficient it is, and it is also being used by Perplexity AI and Fireworks.ai under the hood.
That’s why you’ll often see it pop up in lists of best AI inference models for high performance, and also makes it a strong contender on the list of best free AI models for developers.
Since it’s open-source and optimized for real-time use cases, people are using it in everything from chatbots to backend reasoning engines. You get solid results without needing a cluster of A100s. It's become a go-to in production setups where latency actually matters.
Unlike some heavier models that are tough to scale, Mistral 7B runs super well even on a single GPU setup.
▶️It uses things like Grouped-Query Attention (GQA) and Sliding Window Attention (SWA) under the hood to speed things up while keeping the quality high. That means faster responses, less memory usage, and cheaper infrastructure bills.
Here’s how you can use it using Hugging Face transformers:

Screenshot of using Pieces Copilot to learn how to use Mistral 7B using Hugging Face transformers.
Pros: Fast, accurate, runs well on single GPUs, no need for crazy infra, works well for real-time apps, open weights
Cons: Still needs some tuning for niche use cases, not as "smart" as GPT-4-tier models out-of-the-box
Mistral 7B is great for building AI assistants, summarization bots, or just experimenting with ideas that need quick, consistent results.
Granite 3.0
Granite 3.0 is IBM’s latest open-source model family that quietly does a great job, especially if you’re building enterprise-ready apps. The models range from 2B to 8B parameters and are pretty good at tasks like RAG, summarization, classification, and even tool use.
(Btw, did you know that IBM outperforms OpenAI? It was quite wide-opening for me.)
IBM trained it on a mix of 12 natural languages and over 100 programming languages, which makes it flexible enough for global use and dev-specific tasks. Plus, they’ve added safety layers to reduce bias and toxicity, which is always good if you’re shipping something for actual users.
What’s nice is you don’t need any special setup to try it out. Granite models are available on Hugging Face under Apache 2.0, so just pull them down and go.
Here’s a quick example of how you can use it in your app:

Screenshot of using Pieces Copilot to learn how to use Granite 3.0.
Pros: Granite is open-source and license-friendly, and the only model that I know of which is enterprise-grade but also dev-friendly.
Cons: Even though you can run it anywhere, it still needs a good GPU for larger models and you need to do light fine-tuning for domain-specific stuff.
The good part of Granite is, you can plug it into anything: chatbots, documentation assistants, internal dashboards, or even LLM-powered CLI tools. If you want more control, you can fine-tune it on your data or wrap it in a simple API for your team.
Final thoughts
We’re truly in the golden age of AI models. Whether you’re building side projects, prototypes, or production-ready apps, there’s a model out there that fits your needs and is easy to use (thank god I don’t need to put my Maths skills to test anymore).
I don’t believe there’s a “one model fits all” — I pick and choose based on the task, and I’d encourage you to do the same.
Use Claude 3.7 Sonnet when you want to code, GPT-4o when you want to get creative, Mistral 7B for lightweight inferencing, and Granite 3.0 when you’re working with enterprise-grade apps.
My best advice is that you don’t need to know every single model out there (nobody does), but staying curious and experimenting with tools that remember the context and reduce context switching is the best way to stay ahead.
Download Pieces – it’s free!
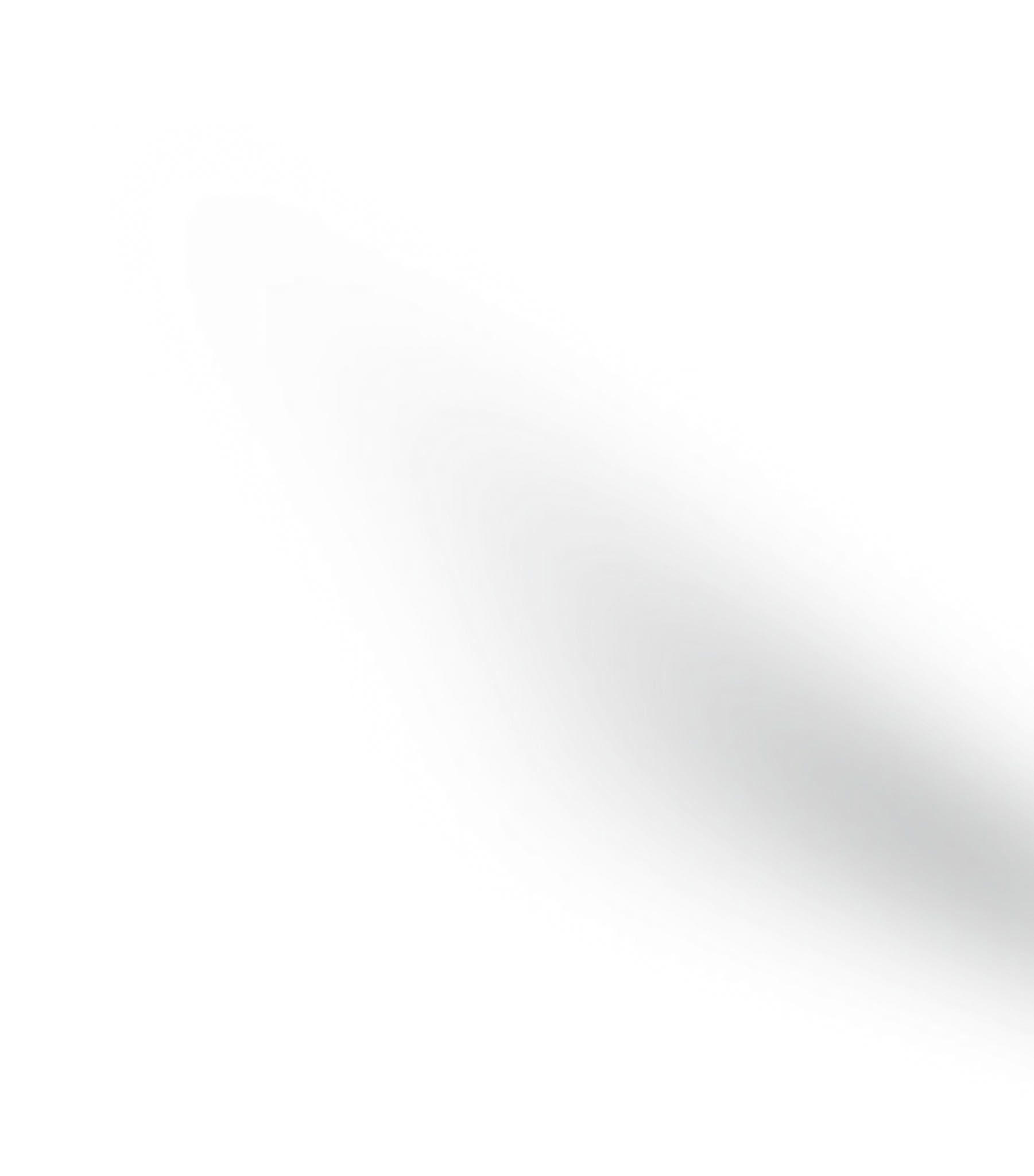